Artificial Intelligence (AI) is transforming countless business practices and automating decision-making processes. In addition to that, it often improves the performance of humans tasked with the same assignment. We have seen this in various fields of logistics, customer care, planning and insurance. However, healthcare seems to be that one domain where the road to AI remains quite bumpy. This post outlines several aspects of AI in healthcare including some examples, its pros and cons and its future outlook.
Why using AI in healthcare?
Systems relying on AI can often use subtle features or detect statistical connections in datasets that human brains are not equipped for. This information enables them to make better decisions and only focus on relevant details related to the patient. Some interesting examples can often be found within the Computer Vision (CV) area. CV focuses on extracting information from pictures and/or videos. Within the medical domain, this would include various types of scans
Tumour detection & measurement on CT or X-ray scans
Even today, software used by radiologists can analyze CT-scans and detect potentially cancerous lung nodules. This enables radiologists to pay extra attention to these specific locations and have properties such as lengths and volumes to be measured automatically. Different kinds of detectors can be built for different diseases. Firstly, major organs such as heart, liver, spleen, kidney, bladder or lungs can be detected and differentiated from the surrounding tissue. Then, abnormalities found within these organs such as tumours, cysts, hematomas, abnormal growths or shapes can be identified and measured. This way, different types of diseases can be automatically detected, increasing the speed and accuracy for radiologists to read these scans.
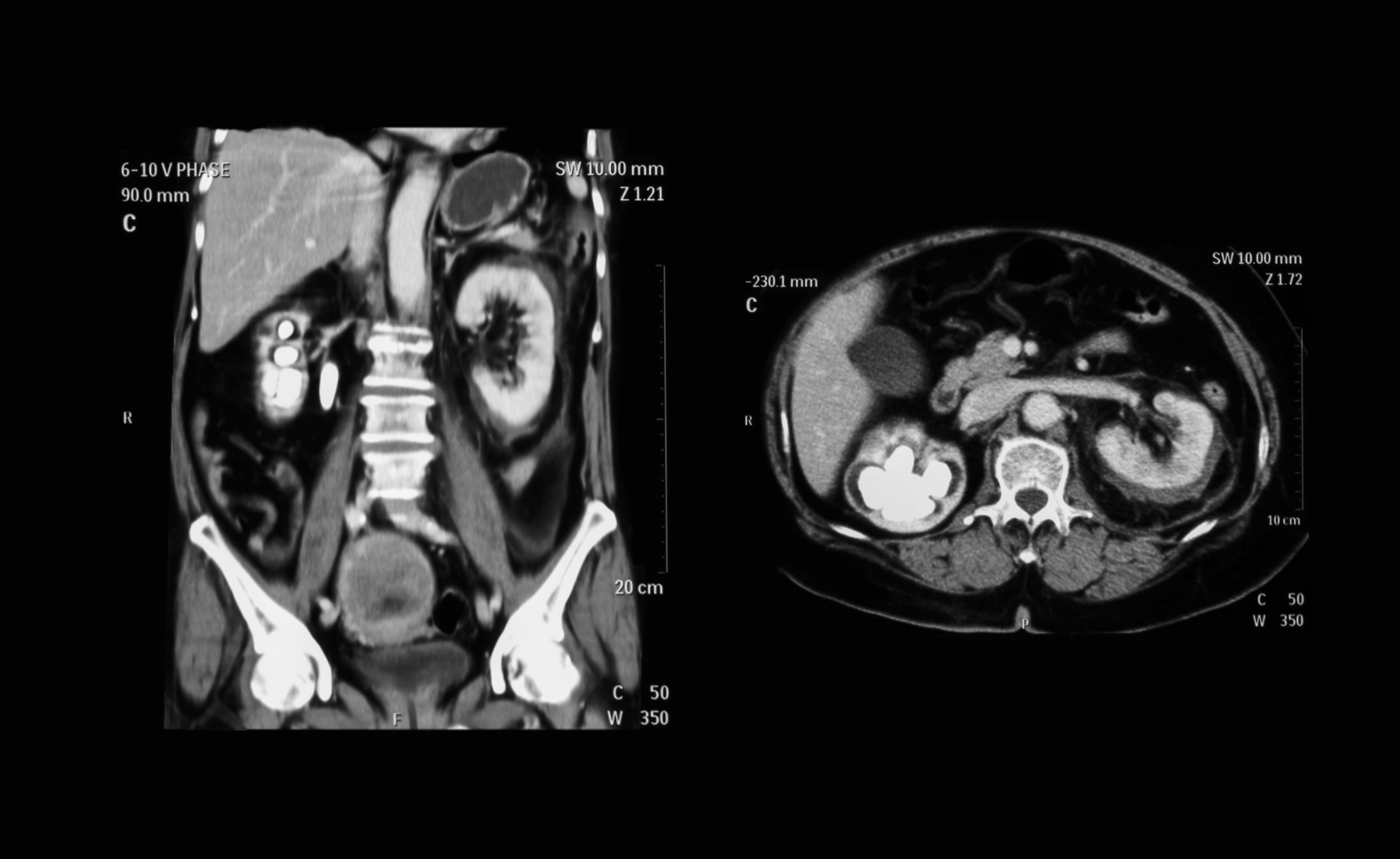

Fetal head circumference measurements
It is common for women to get an ultrasound scan during their pregnancy. The purpose of this scan is to keep track of the fetal development, which includes the measurement of the fetal skull circumference. It requires an expensive device and a highly trained specialist to perform this task. Fortunately, software that is capable of automatically measuring these things has been developed. This software can work with low-quality data obtained by cheaper ultrasound devices and less trained operators. This makes it easier to introduce this service to developing countries, thereby reducing childbirth complications.
Tasks in dermatology – melanoma classification
Have you ever had concerns about abnormal growth of moles on your body? Usually, it would take a GP to look at it. A GP could further refer you to a dermatologist if they think it is alarming. Australian scientists have developed a system which can automatically rate moles based on pictures. According to their paper, it was more accurate than an average dermatologist.

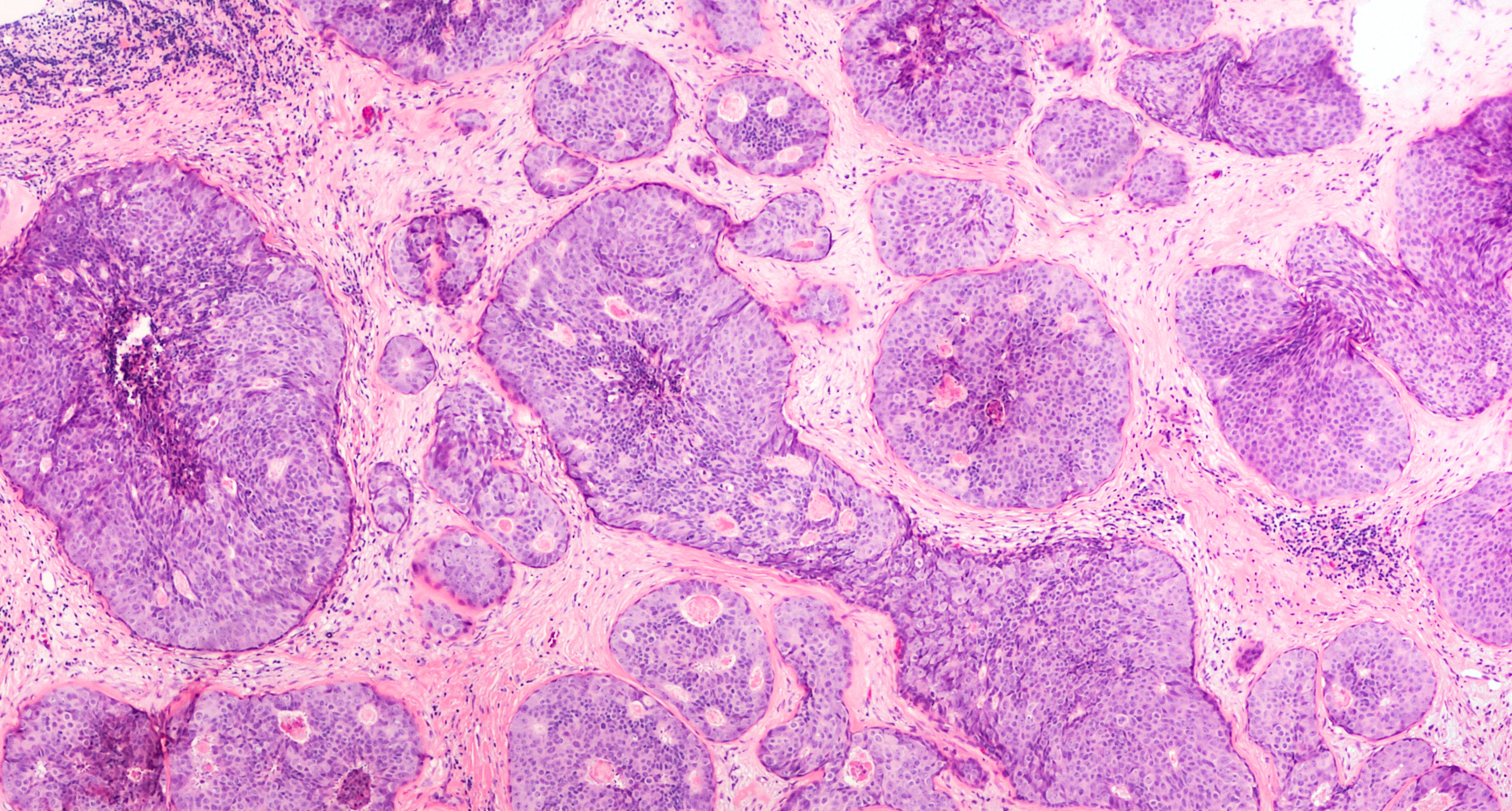
Reading of pathological slides
In the Netherlands, women between the age of 50 to 75 years old are encouraged to participate in breast cancer screening every two years. If an abnormality is found, it can be surgically removed. The removed tissue is then inspected for malignant tumour cells. This can be done by cutting thin slices of the tissue and putting them under a high-resolution scanner to digitize them. Due to the enormous size of these images, pathologists are limited to inspecting only a few areas of these tissue slices. It means that they are not able to identify all of the bad cells. In contrary to this, a computer system is capable of counting cancer cells with more accuracy and speed by reading the entire scan.
But what is the problem?
The pressure to comply with each of the requirements for using algorithms in healthcare applications is incredibly high. It does not come as a surprise, given the nature of this field, which requires the utmost accuracy in terms of patient treatments. Furthermore, strict regulations play significant roles in this industry. So it is crucial to make an exceptionally reliable system.
Currently, there are a couple of roadblocks in implementing AI solutions to replace a doctor’s judgement:
- Biased training sets. Think of a system trained on MRI scans based on one particular brand of scanner, or based on scans of only middle-aged people. The performance of the system among patients within this group could be regarded as excellent. But when it comes to scanning a young person with a different MRI scanner, the performance could degrade drastically.
- Confidence in predictions. You cannot simply send someone home if you are only 51% sure that it is not cancer, but perhaps you do if you are 99.9% sure. For current deep learning-based systems, getting these accurate estimations is still difficult. However, this problem is acknowledged within the community, so research is currently being done in this area.
- The societal and legal status of AI as (substitute for) a physician. A patient might be more forgiving when a doctor makes a mistake (they are only human after all!) than a computer. Hence, most systems are currently used as an additional source of information supporting human physicians in making the final diagnosis. Furthermore, there is an ethical debate going on about how independently an AI system should be allowed to operate and make decisions.
Road ahead
It is difficult to predict what will happen in the future, but the general assumption implies that the role of a doctor will change. Doctors will focus more on complex cases, making their work more exciting and challenging. While “simpler” and tedious jobs that require a lot of precision will be offloaded to software. In the case of radiology, the amount of scans and photos that are being made continue to increase rapidly every year. Which is in disproportion with the numbers of radiologists trained to read them. This makes it necessary to increase the usage of AI to keep up with the situation.